Keyphrases:
RNN, Recursive Neural Networks, MV-RNN, RNTN
description: This set of notes discusses and describes the many variants on the RNN (Recursive Neural Networks) and their application and successes in the field of NLP. —
Recursive Neural Networks
benefit:
- recursive structure
- input sentences of arbitrary length
Let’s imagine we were given a sentence, and we knew the parse tree for that sentence.
A simple single layer RNN
We now take $h^{(1)}$ and put it through a softmax layer to get a score over a set of sentiment classes. In the case of positive/negative sentiment analysis, we would have 5 classes, class 0 implies strongly negative, class 1 implies negative, class 2 is neutral, class 3 is positive, and finally class 4 is strongly positive.
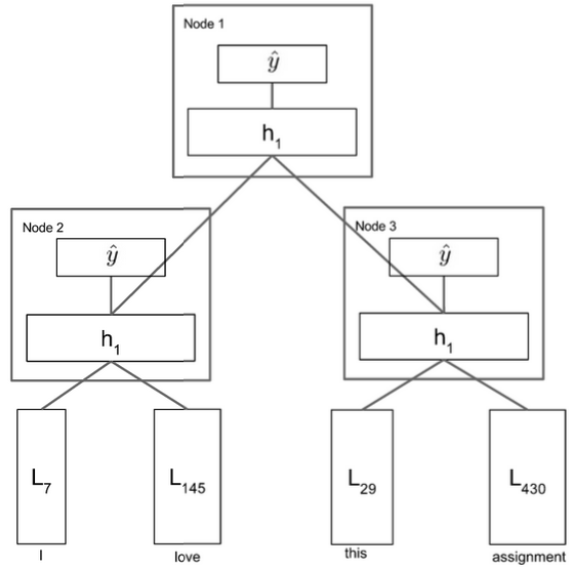
Now lets take a step back. Is it naive to think we can use the same matrix W to concatenate all words together and get a very expressive $h^{(1)}$ and yet again use that same matrix W to concatenate all phrase vectors to get even deeper phrases.
Syntactically Untied SU-RNN
There is no reason to expect the optimal W for one category of inputs to be at all related to the optimal W for another category of inputs. So we let these W’s be different and relax this constraint.
SU-RNN的主要思想是用PCFG先给句法树种的每个节点和词打上标签。然后DT-NP拼接的时候用一种W,VP-NP拼接的时候用另一个W,这样可以提高模型的描述能力。
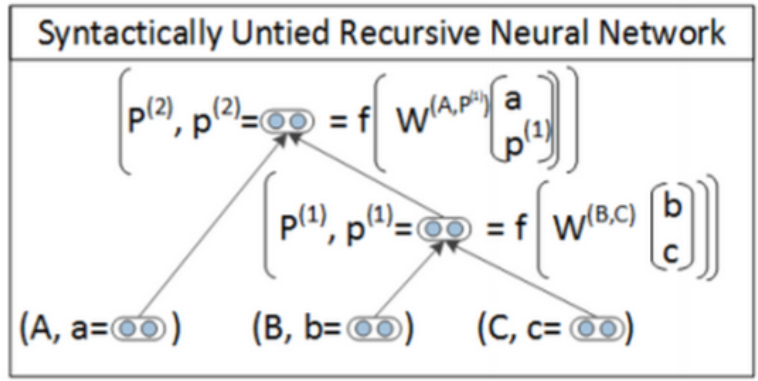
The only major other difference in this model is that we initialize the W’s to the identity. This way the default thing to do is to average the two word vectors coming in.
SU-RNN比之前的模型有了一定的改进,但是还解决不了修饰词的问题,例如very这个词会本身就会使得另一个词的词意变强,通过SU-RNN的这种线性插值的方法无法让某个向量去放大另一个向量。
MV-RNN’s (Matrix-Vector Recursive Neural Networks)
We now augment our word representation, to not only include a word vector, but also a word matrix!
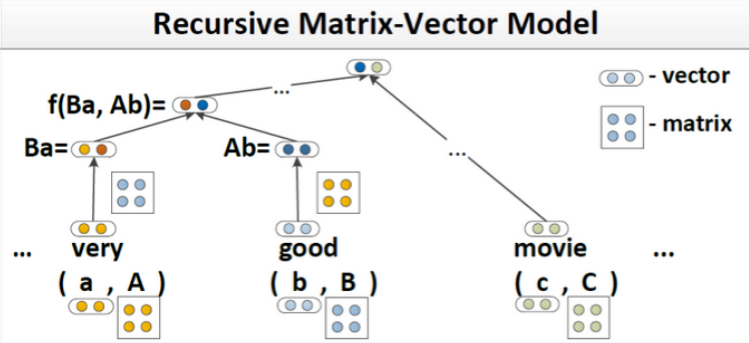
RNTNs (Recursive Neural Tensor Network)
To compose two word vectors or phrase vectors, we again concatenate them to form a vector $\in R^{2d}$ but instead of putting it through an affine function then a nonlinear, we put it through a quadratic first, then a nonlinear, such as:
其中$V\in R^{2d\times 2d\times d}$是一个三阶的张量。 $x^TVx$的计算方式是把张量的每一个分片(张量的一个分片维度是$2d\times 2d\times d$)计算$x^TV[i]x$,最后输出一个$R^d$的向量。通过二次变换实际上两个向量之间进行了乘法类型的交互,而且不需要像MV-RNN一样对每个词保持一个矩阵,参数空间小了很多。

Constituency Parsing
Constituency Parsing is a way to break a piece of text (e.g. one sentence) into sub-phrases. One of the goals of constituency parsing (also known as “phrase structure parsing”) is to identify the constituents in the text which would be useful when extracting information from text.
Constituent
For constituency parsing, the basic clause structure is understood as a binary division of the clause into subject (noun phrase NP) and predicate (verb phrase VP), expressed as following rule. The binary division of the clause results in a one-to-one-or-more correspondence. For each element in a sentence, there are one or more nodes in the tree structure.
Constituency Parse Tree
Interestingly, in natural language, the constituents are likely to be nested inside one another. Thus a natural representation of these phrases is a tree.
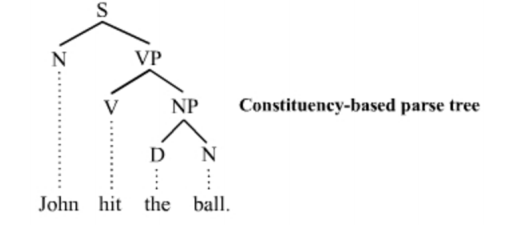